Reflectance October 2020
Quick Updates, Exposing Bedrock by Matt Rossi, and Project Impervious Surface Updates
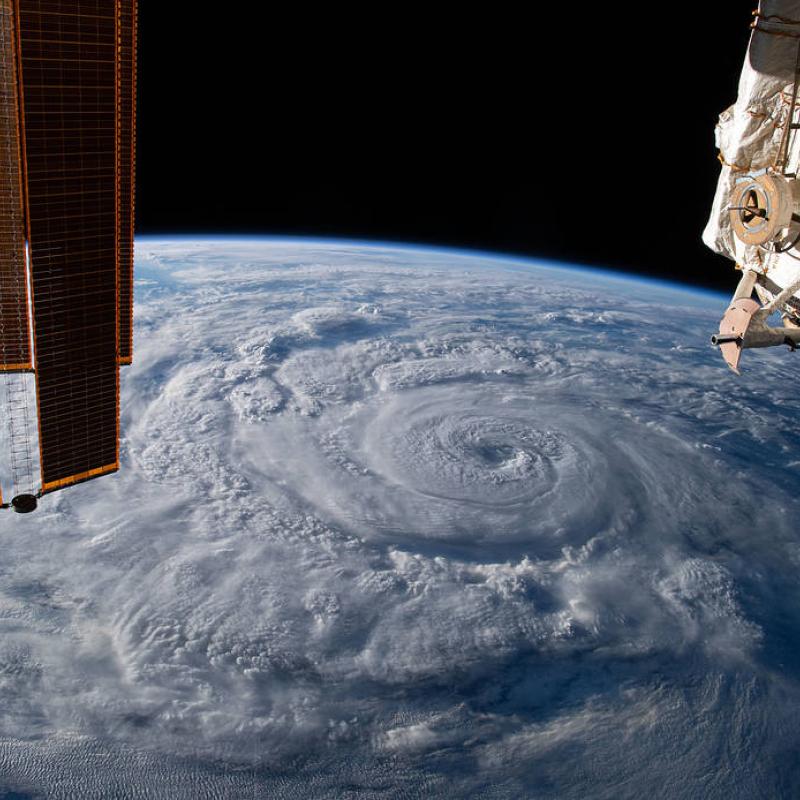
Quick Updates
- It’s official - a collaborative grant, submitted to the NSF Macrosystems Program by Earth Lab, has been recommended for funding. This proposal, headed by PI Jennifer Balch, will explore forest resilience to disturbance, scaling from individual trees to large swaths of forest, using open-source UAS, NEON, and Landsat data. Focusing on the western U.S., these findings can help improve forest system recovery and better understand compound disturbances.
- During the summer/fall months, Earth Lab’s Fire, Extremes, and Health teams were often asked to give perspective on current fire events. By the end of October 2020, following a particularly rough fire season for Colorado and the West, members of Earth Lab were featured in media 35+ times, from interviews to providing research as evidence. Example: "We’ll Have to Learn to Live With Smoke. Here’s Why." by Julia Rosen for the NY Times, 9/23
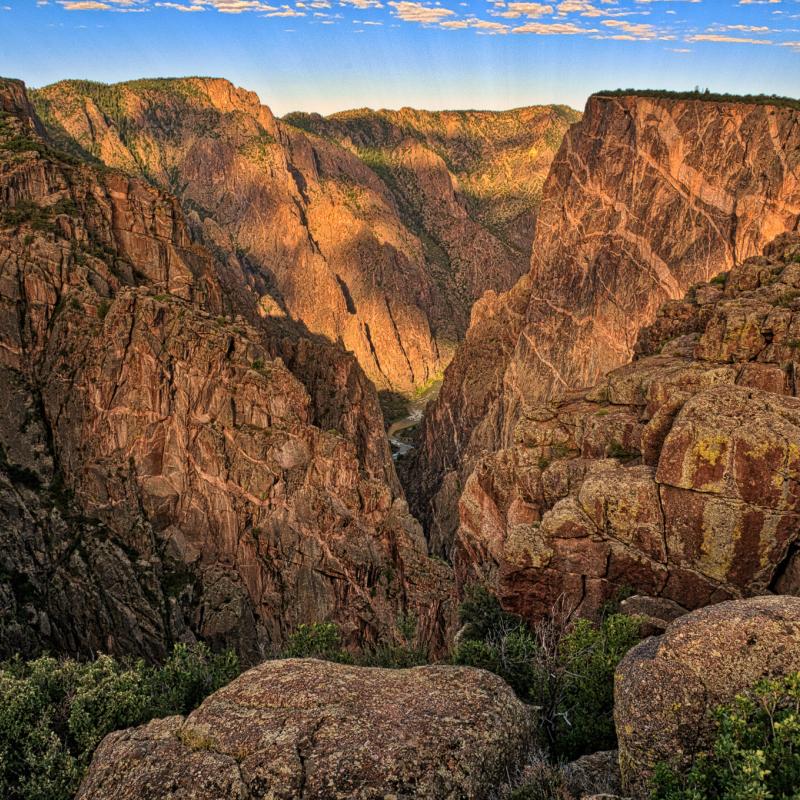
Exposing Bedrock: A Marker of Stability or Instability?
Where Earth is not covered by water, it is usually covered by a life-sustaining mix of mineral and organic matter called soil. Despite the ubiquity of soil, some of the most inspiring landscapes are those where the ‘skin’ of the Earth has been stripped away (think bedrock gorges and glaciated peaks). The stark absence of soil in these settings is what makes them unique, alluring, and beautiful. Understanding how they formed requires thinking across at least seven orders of magnitude—a landslide can occur in a few minutes while 1-m of soil can take many millennia to form. Knowing how and where soil persists is essential to ecosystems, agriculture, and natural hazards.
The loss of soil is often attributed to disturbance, but can patchy soil cover be stable in the statistical sense? In Project Erosion, we are pondering these questions through the lens of the soils, tors (aka isolated bedrock outcrops), and rocky cliffs of the Colorado Front Range below the glacial limit. Incised canyons are dominated by steep, rocky cliffs with trees seemingly growing on rock. Elsewhere, soil cover is higher, yet soils are still very thin and patchy. Our research is showing that soil patchiness is a stable feature of the landscape driven by relatively small differences in the pace and pattern of bedrock river incision.
Can we relate fractional soil cover to process? Insights from numerical models that treat trees as agents with simple rules for sapling recruitment, growth, and death suggest we can. We ground these model results in reality using drone-based photogrammetry and multi-spectral lidar data to characterize how bedrock exposure varies as a function of landscape position and time. So the next time you drive up into the mountains, ask yourself why and where soil is absent, and whether the bedrock you see is a stochastic accident or a persistent feature of the landscape.
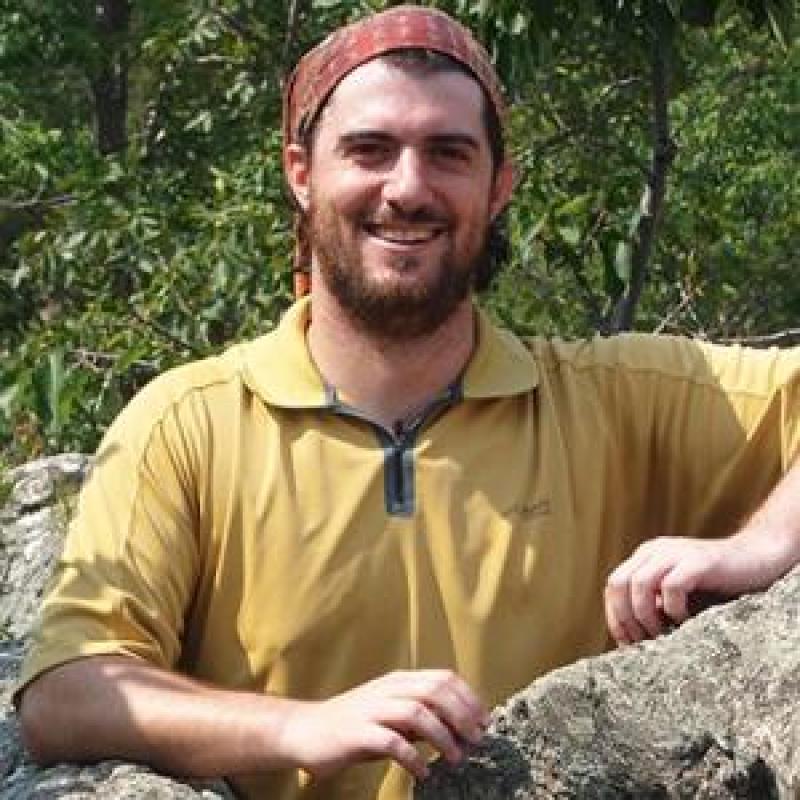
Matthew Rossi is a Research Scientist in Earth Lab who primarily works on Project Erosion and Project Extremes. His recent work focuses on orographic controls on rainfall variability, vegetation dynamics, soil production, runoff generation, and river incision.
Impervious Surfaces - Project Updates
Earth Lab’s remote sensing scientist Joe McGlinchy, in collaboration with Brian Muller (ENVS), has been working with a fully convolutional neural network (FCNN) known as UNet in order to classify impervious surfaces from satellite imagery. Their success in this area has implications for mapping urban sprawl, surface water run-off, and the urban heat island effect.
UNet is a FCNN architecture which has been proven to be a useful network architecture for pixel-level classification from one to many classes. The impervious surface class is represented by many distinct classes, so the team wanted to see if UNet could be used as a one-shot tool to classify impervious surfaces. DigitalGlobe’s Worldview-2 system includes 8 spectral bands within the visible-to-near-infrared region and provides a nominal 2-meter pixel size. Outputs from the UNet FCNN, along with a dataset produced by a land cover algorithm from the same scene, were used to compare the UNet results across tiles for a test dataset across central Denver, Colorado. They observed, on average, better segmentation performance from UNet and much improved segmentation in residential areas. Similarly, the team found satisfactory segmentation in areas outside of the ground truthed area.
High resolution maps of impervious surfaces are useful at the source image resolution and can be aggregated to levels relevant to management activities, such as parcels or blocks. Ideally, these maps can be produced from any image from any time at any location to understand the spatiotemporal distribution of the surface, making generalization a direction for future work.
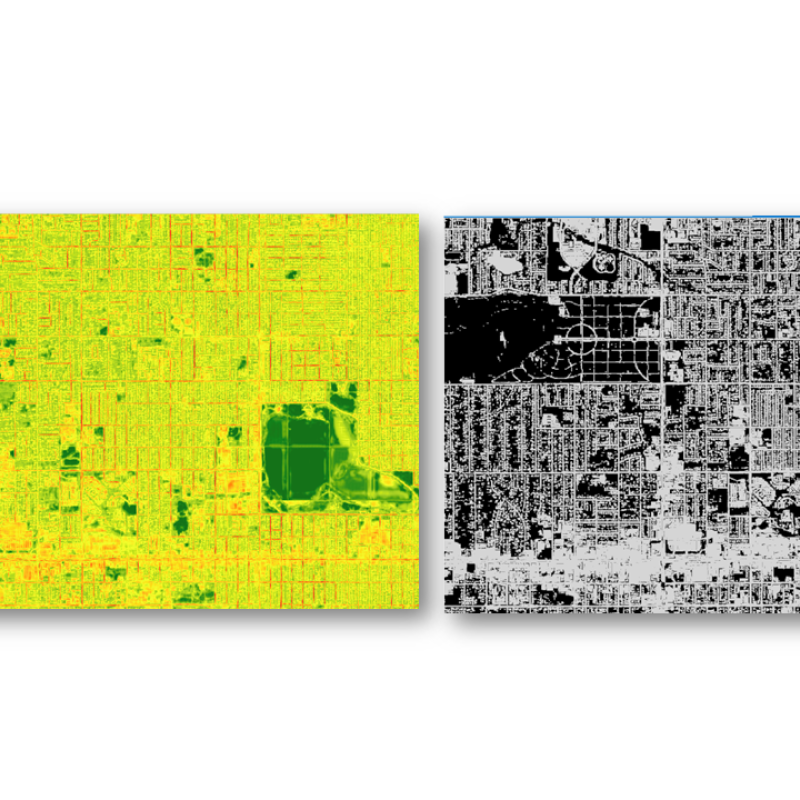
(left) A map of impervious surface probability output from the trained UNet model thresholded (right) to represent the impervious surface class.