Wildfires: A Rapidly Growing Problem
How can we quantify health impacts from different types of air pollutants and different types of fires, and how can we improve public policy to reduce these health impacts?
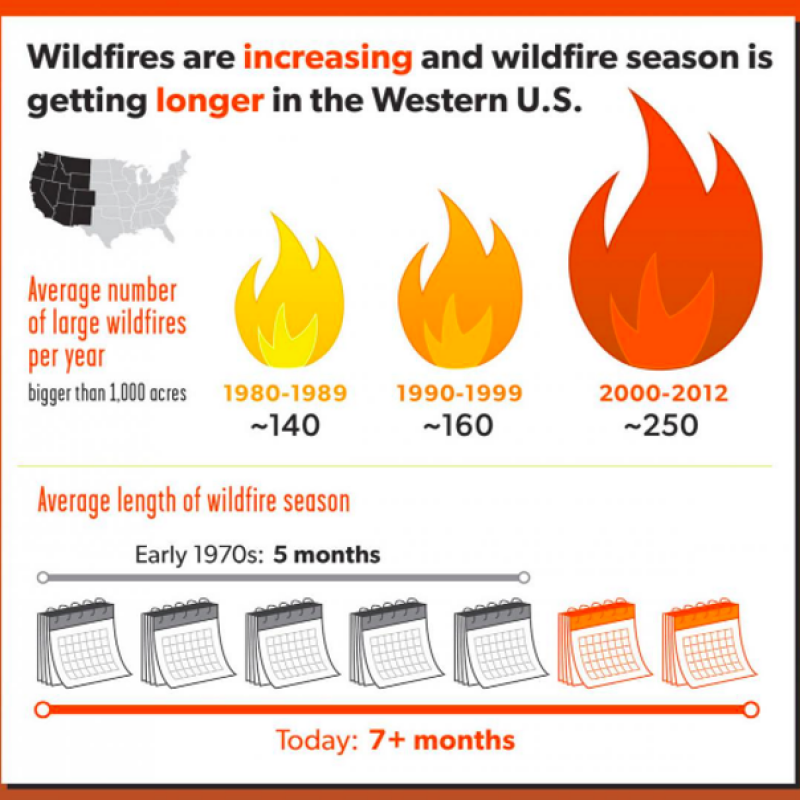
The intensity of wildfires in the western US has been increasing both in terms of acres burned and economic impact. This situation means different things to different people: policymakers debate the best methods to mitigate the costs, environmental advocates argue that we need to take action against climate change, and people living in areas recently impacted by fire feel a gamut of emotions.
One aspect that we don’t often hear about is the impact of the resulting air pollution on human health. Whether we are breathing in fine particulate matter or ground-level ozone, both short and long term exposure can degrade our health. This problem poses many questions. For example, how can we quantify health impacts from different types of air pollutants and different types of fires, and how can we improve public policy to reduce these health impacts?
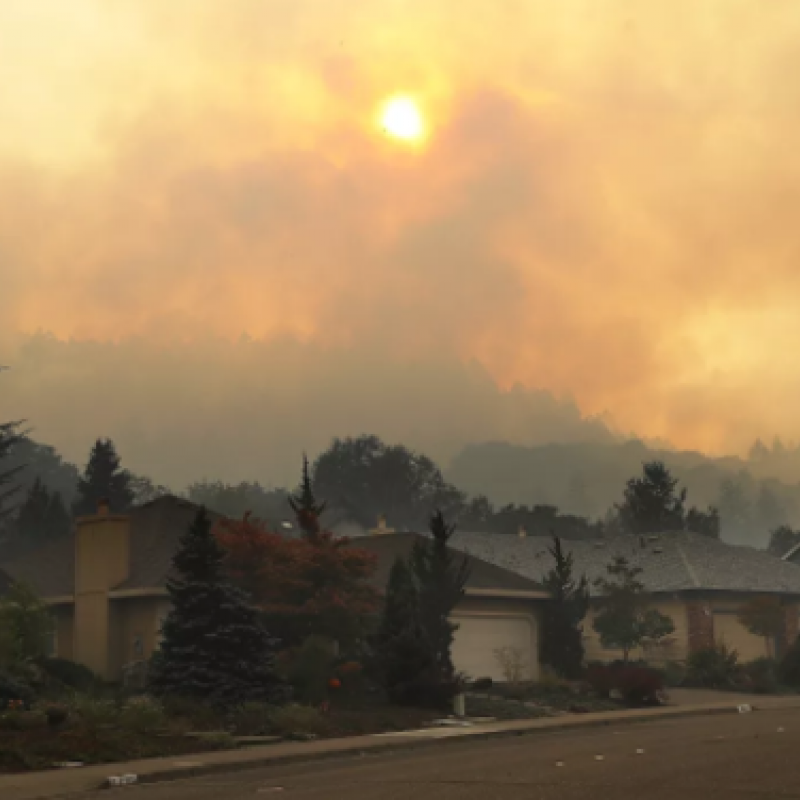
Air pollution from wildfires
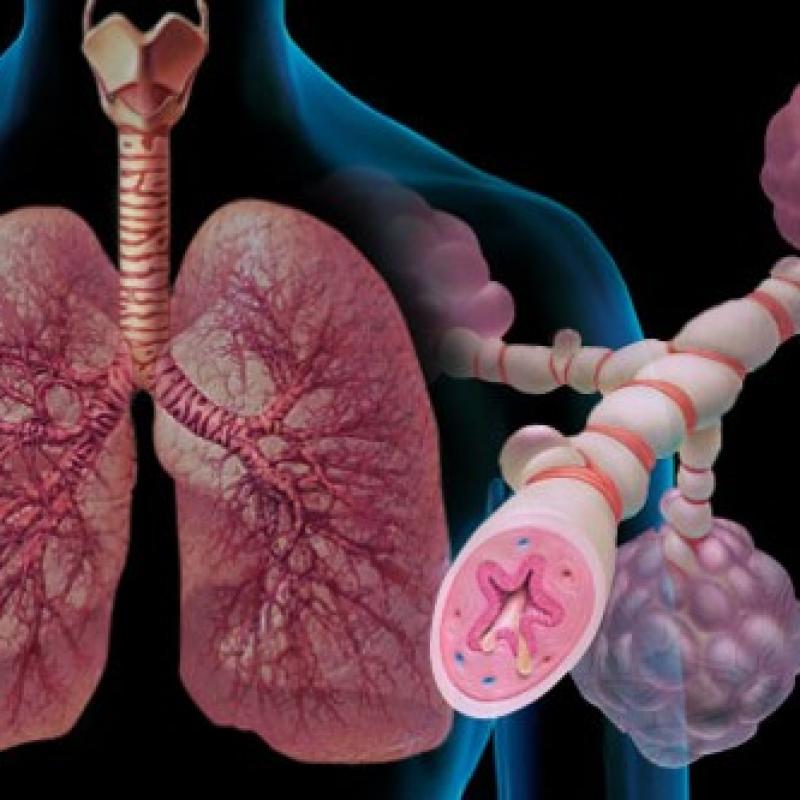
Asthma in the respiratory system
Fire and People: Researching the Health Impacts of Wildfires
Four months ago, I began working with the Environmental Health team in Earth Lab. Our team consists of University of Colorado professor Colleen Reid, post-doctoral researcher Melissa May Maestas, graduate student Gina Li, and an undergraduate intern (me). This interdisciplinary team is currently working on two projects to investigate the impact of air pollution from wildfires on human respiratory and cardiovascular health. Both projects require two stages of analysis: modeling air pollution from the fires and then modeling the health responses.
Dr. Reid and I are near the end of finishing a study of the health effects of PM2.5 (fine particulate matter) and ground-level ozone from the northern California wildfires in 2008. This project is the frontrunner in looking at the effects of ozone from wildfires on human health.
For the Data Scientists: a Statistical Smorgasbord
The air pollution model is borrowed from one of Dr. Reid’s previous papers, for which her team used 10-fold cross-validation to choose the statistical algorithm that resulted in the best predictor of PM2.5 in the air quality data. The study concluded that a generalized boosting model (GBM), resulted in the best air quality predictions.
The health response model uses regression to calculate the relative risk of hospitalization for ten different respiratory and cardiovascular health responses associated with an increase in air pollution. The analysis explores the effects of PM2.5 and ozone, individually and mutually adjusted for each other. We compared the time periods before, during, and after the 2008 wildfires. Our study area consisted of the Sacramento Valley and San Joaquin Valleys in north-central California.
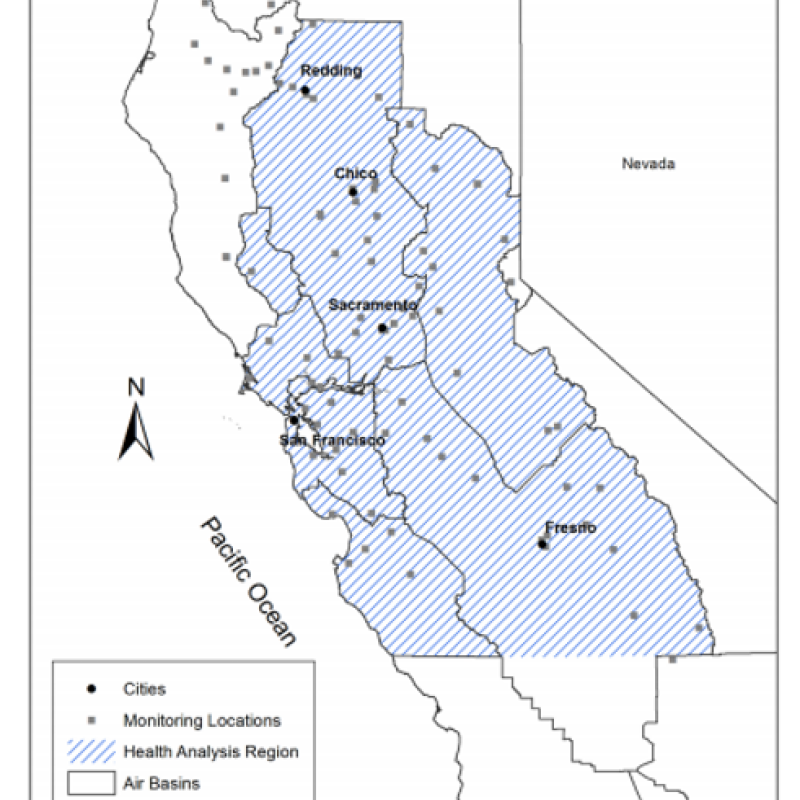
One important statistical question is how to choose the type of discrete regression that best fits the health data, either Poisson regression or negative binomial regression. Because the type of regression depends on the distribution of the health outcome variables, this boils down to determining what kind of distribution fits the data most closely. The hospitalizations are count data, and the standard distribution for count data in epidemiology is the Poisson distribution. However, Poisson regression is not the most accurate if the means and variances of all of the data are not extremely close (the same, in theory). If the variance of a variable is greater than the mean, then we say the data is overdispersed. Overdispersion can arise when data are either not identically distributed or not independent, which often are related and difficult to distinguish. One intuitive reason that some of our data may fit both of these classifications (although we can’t be sure whether one, the other, or both, is most accurate) is that neighboring ZIP codes often share many societal and physical characteristics (such as air pollution levels) that would affect the number of hospitalizations from a given increase in air pollution. For instance, low incomes are often clustered geographically, i.e., poor people tend to live near each other. Low income and lack of health insurance often result in more emergency department visits instead of routine checkups when an air pollution event (such as a fire) occurs, which would then make it more likely that our model would be making biased predictions based off of non-independent, extreme health response data from neighboring ZIP codes.
Another type of discrete regression is the negative binomial, which “adds a multiplicative random effect to represent unobserved heterogeneity”. The negative binomial regression is more appropriate when the data is overdispersed.
To determine whether our data is overdispersed, or at least whether a negative binomial regression is more appropriate than a Poisson regression, I have been researching standard model selection methods. A fairly simple method is the deviance goodness-of-fit test. The deviance of a model indicates how much worse it fits the data than the saturated model, which would have one parameter per observation and thus fit the data perfectly. I am currently in the process of applying these tests to our data.
Policy as a Solution: Our Second Project
One tool that is currently used to reduce the future intensity of wildfires is ‘prescribed fire.’ Prescribed fires are set to reduce the danger of natural wildfires and to fulfill the ecological needs for fire, such as cleaning the forest floor, killing disease-carrying insects, and allowing some plant species to reproduce. The fires are set in controlled ways and generally burn at lower temperatures and on smaller scales than naturally-occurring wildfires. As a result of their controlled nature, the air pollution levels of prescribed fires are normally much lower than those of wildfires.
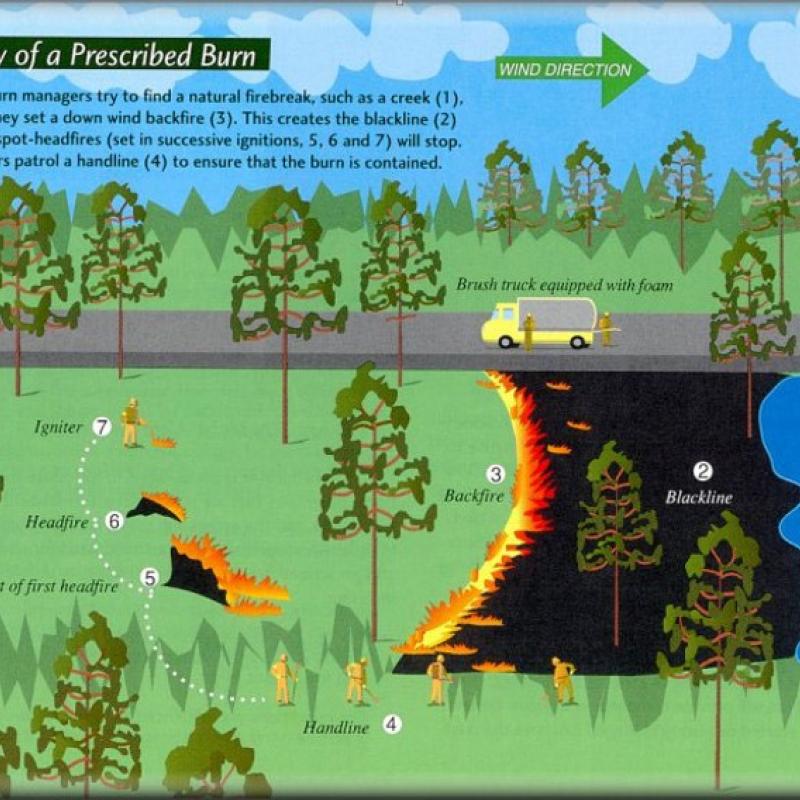
We know that because of the differences in temperature, geographic size, and timespan of these fires, each type of fire pollutes the air differently, both with varying chemical compounds and absolute amounts of each pollutant. However, there’s one critical issue that we don’t understand: whether wildfires and prescribed fires have differential health effects.
Our group in Earth Lab is investigating the differential effects of wild and prescribed fires on the health of people in 11 western states from 2008-2014 (see map below). The team is just in the beginning stages of this project now, but after our analysis is done, the eventual findings of this project will be used to inform state air quality managers and public health departments about how best to allocate their resources and notify citizens when air pollution levels are dangerously high, through our partnerships with the Western States Air Resources (WESTAR) Council and the Centers for Disease Control and Prevention’s Environmental Public Health Tracking Network (EPHTN).
Prescribed burning is understandably controversial. Some stakeholders advocate more prescribed burning and some argue for less, with solid arguments on both sides. Prescribed burning releases smoke and to truly decrease the risk of wildfire, a large acreage needs to undergo prescribed burning, thus it could lead to more regular, but lower-level air pollution impacts. Prescribed fires also have the potential for getting out of control, but without prescribed burning, we risk more catastrophic wildfires, which can lead to very high amounts of air pollution on an irregular basis. With reliable data on the health impacts of different types of fire, policy makers will be able to make more informed decisions about fire mitigation and provide much more specific information to civilians about how best to reduce the risks to their health. For example, they might be able to implement a data-driven text- or email-notification system to alert people about the air pollution and resulting health risks of outdoor physical activities.
Lots and Lots of Data
Much of my work in the fall consisted of finding and sorting through appropriate data for our team’s analyses. One of the coolest things about earth data science is that you can use various data to create accurate models and account for confounding factors. Wildfire-health analysis requires both environmental and health data to model the exposures from air pollution and the health responses. Thus far, I have explored or used the following data sets:
- Hospitalizations and Emergency Department counts from 11 western US states: our measure of health response to air pollution
- Census data (socioeconomic confounding factors): controls for health response by ZIP code and is useful in analyzing differential health impacts based on race, sex, age and socioeconomic status
- Estimates of smoking rates by geographic area: a confounding source of air pollution that degrades people’s health
- Meteorological data from NOAA: used in the air quality model to control for different weather and climate factors
- Imagery from geostationary satellites: used in the air quality model to track fire smoke and corroborate ground air quality and meteorological monitors
- US state and federal highway spatial files and traffic counts: another confounding source of air pollution
- PM2.5 (airborne fine particles) measurements from the US EPA: one measure of air pollution from fires -- see (below) map of air quality monitoring stations in the western states
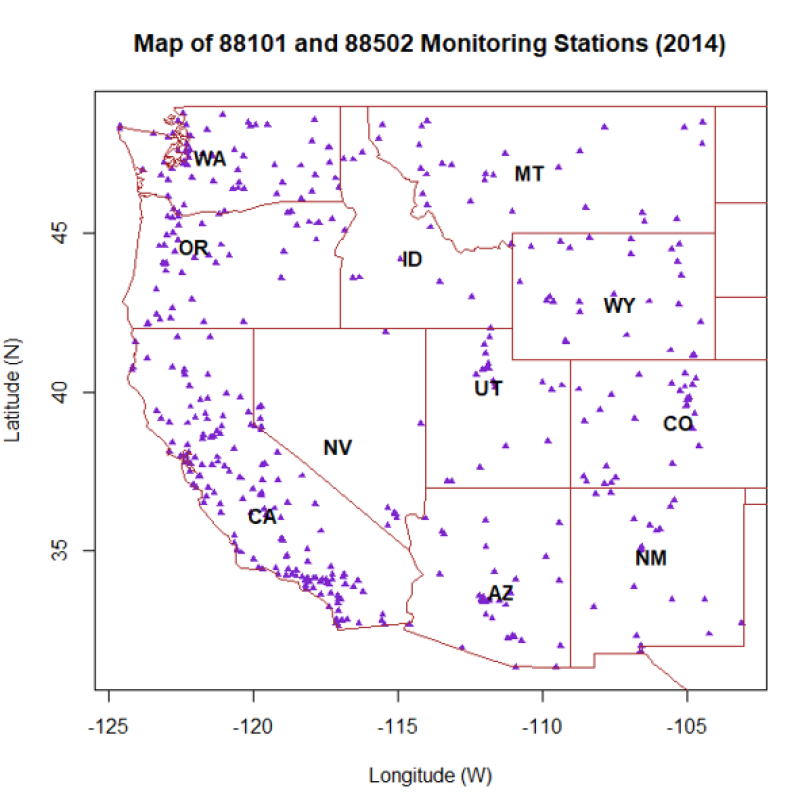
Both the 2008 California fire and 2008-2014 western US air quality modeling and health response analyses require even more sets of meteorological and earth imagery data, but the range of necessary data types is apparent in the list above. A significant challenge in our work is collecting sufficiently accurate and large amounts of data. For example, there are a limited number of reliable air quality monitors, especially in the western states (see map above). From the health side, it is very difficult to gain access to confidential hospital data, and some information, such as smoking rates, are most often published in aggregate at spatial resolutions coarser than the ZIP code level (often at the county level). Thus, we rely on techniques and data generated and collected by other researchers in fields ranging from atmospheric science to epidemiology to supplement our models and invigorate our study.
Concepts in Modeling
Moving forward with the 2008-2014 project, our team will be using some interesting techniques in statistical modeling, which are intuitive enough in theory, if not always in practice. The main ones are methods to account for exposure measurement error in the health analysis, assessing distributed lags, case-crossover analysis and effect modification by categorical and continuous variables. The following paragraphs describe each of these methods.
Because of sparse air quality monitoring data and the heterogeneity of air quality over large regions, researchers often create models of air pollution over space and time. These are models, not observations. Thus, there is exposure measurement error in using these to estimate human exposures to air pollution. If we try to predict health outcomes with these modeled exposure estimates, without taking into account the uncertainty in the model (exacerbated by uncertainties in the direct measurements), the estimates may be inaccurate.
Comparing health responses to fire occurrences requires accounting for the time it takes for people to feel the effects of smoke and then make it to a hospital. Variation here depends on the time it takes for smoke to spread to where people are, people’s susceptibility due to age, sex, or a pre-existing respiratory or cardiovascular health condition, and people’s willingness and ability to go to and pay for a hospital visit. Because not every person will experience the same lag time between exposure and health outcome, we often estimate a combined effect for all lags within a certain time period to understand the total effect of a given day’s air pollution on that health outcome across the population. To recognize the full impact of each fire, we can mark each of the days in the week following the pollution event as lag1, lag2, lag3, and so on. Analyzing these distributed lags allows us to observe not only the full effect of high air pollution during each fire, but also the time it takes for different health outcomes to develop given certain types and amounts of air pollution.
Accounting for sociodemographic factors, which might otherwise alter the observed effect of air pollution from fires on human health, can take several forms. In our studies, we use case-crossover design and effect modification. In case-crossover design, each individual serves as their own control, removing the need to control for sociodemographic data that does not change in an individual over a short period of time (such as gender, age, smoking status, and socioeconomic status). This analytical method can be used when you are looking at short-term exposures, over a few days, for example. For each hospitalization in the data set, we compare the air pollution level on the day (and corresponding lag days) that someone went to the hospital for a cardiorespiratory event to the air pollution level on control days. Control days match the same day of the week as the hospitalization in the same month as the hospitalization, thus adjusting for the fact that there are weekly and seasonal trends in hospitalizations. Then, the only change likely causing the individual’s hospitalization is the air pollution they were exposed to.
When we are looking at exposures that occur over a longer time period, we need to consider the effect modification by sociodemographic factors on the aggregate health burden. This means including either continuous or categorical versions of US Census variables in the model regressions. If we observe differential associations between wildfire air pollution and cardiovascular and respiratory health outcomes by different categories of sociodemographic variables, we would be identifying populations that are more or less affected than others by wildfire smoke. This identification of vulnerability (or lack thereof) is helpful in policymaking.
The Big Picture
Environmental and social problems are inexorably tied to each other and to technology in today’s world. With increasing amounts of global data and analysis tools, we have the opportunity to closely study the interactions of humans and Earth systems, inform policy making, and effectively pave the way for a more sustainable and healthy future. In other words, the future of this field is bright.
Sources:
https://www.mnn.com/health/healthy-spaces/blogs/california-wildfires-creating-unprecedented-air-pollution-crisis
https://www.webmd.com/asthma/ss/slideshow-asthma-overview
http://pubs.acs.org/doi/10.1021/es505846r
GBMs: https://support.bccvl.org.au/support/solutions/articles/6000083212-generalized-boosting-model
https://www.ncbi.nlm.nih.gov/pubmed/26231012
https://onlinecourses.science.psu.edu/stat504/node/162
Techniques to counter overdispersion and zero-inflation: http://data.princeton.edu/wws509/notes/c4a.pdf
http://www.fire.ca.gov/communications/downloads/fact_sheets/TheBenefitsofFire.pdf
https://pagamecommission.wordpress.com/2015/09/02/prescribed-fire-and-wildlife/