Reflectance April 2021
Quick Updates, Training Climate Scientists, Graduate Student Team
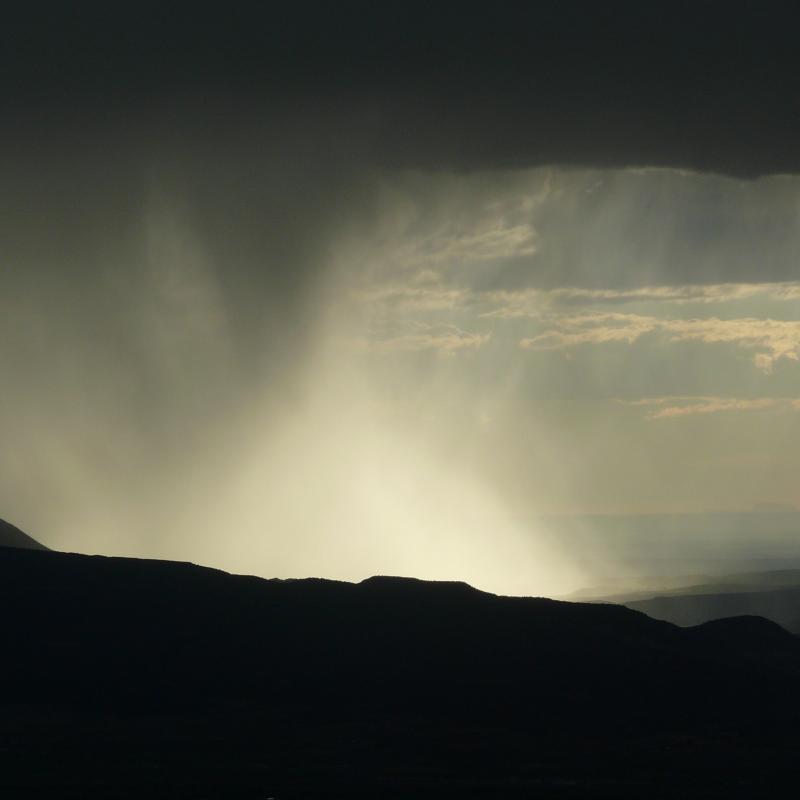
Quick Updates
- As of May 3rd, we are proud to announce the full launch of our new Earth Lab website! This has been a significant effort for our team and we are excited to share it with you. Thank you for your enthusiasm for our work! If you have comments about the site or feedback on how we can make it better, please email earth.lab@colorado.edu.
- The Earth Analytics Education team recently received a $574,000 grant from the SLOAN Foundation to build the pyOpenSci community over the next two years. pyOpenSci is an organization that creates processes, standards, and an inclusive and diverse community around the development and maintenance of Python packages.
- NSF featured one of our Earth Data Science Corps projects working with Tribal communities to utilize COVID-19 data. Read about it here.
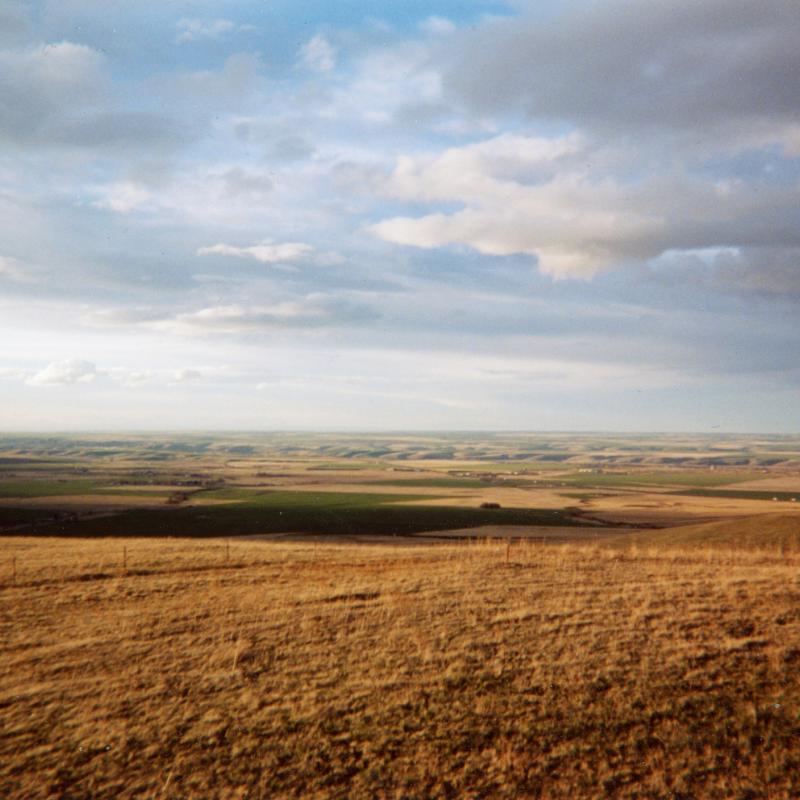
Earth Lab Trains Climate Scientists in Data Skills
From severe wildfires to reduced agricultural yields, the impacts of climate change on the central United States are becoming increasingly alarming. In tandem, the volume of Earth data available to understand, predict, and respond to these impacts is growing rapidly. In this context, equipping climate stakeholders with data skills has never been more important. The North Central Climate Adaptation Science Center (NC CASC) and Earth Lab work to train scientists and natural resource managers through data-intensive workshops that cover core scientific programming and Earth data topics.
Teaching the Key Data Skills That Climate Stakeholders Need
NC CASC data skills workshops are aimed at federal and state agency employees, members of Tribal organizations, university researchers, and others who address global environmental change. To determine the most relevant content for these stakeholders, we surveyed nearly one hundred climate adaptation researchers and practitioners to identify their biggest skill gaps, which we integrate into workshops. Upon completing workshops, participants are equipped with new skills enabling them to teach, learn, and co-produce actionable knowledge. In our most recent workshop, participants learned how to use Python to find, process and visualize MACA v2 climate data in the netcdf hierarchical data format - - valuable skills for climate adaptation science.
Free and Open Climate Data Learning Materials Maximize Reach
There has been overwhelming interest in past NC CASC workshops, reflecting a great need for training in data-intensive skills for those in the workforce. To maximize access, workshops are taught using JupyterHub, a cloud-based programming platform that removes the need for software installation or a powerful machine. Our events support fully virtual attendance, with participants in our most recent workshop coming from 15 states across the U.S.
To achieve the broadest reach possible, we publish all training material online. You can find all of the lessons from our recent Climate Data 101 in Python workshop, along with hundreds of others, on Earth Lab's free and open education learning portal. You can also go through the activities from the Climate 101 workshop on GitHub.
View other work by the NC CASC here.
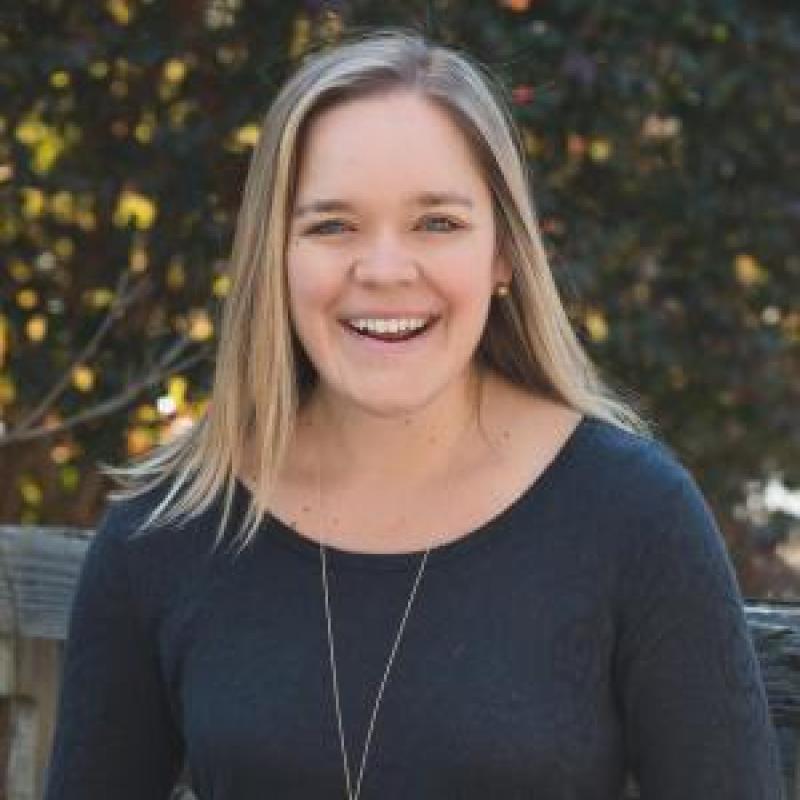
Lauren Herwehe is the Earth Analytics Education Initiative Program Manager. She organizes workshops for the NC CASC. She also manages the Earth Data Analytics - Foundations Professional Certificate and NSF-funded Earth Data Science Corps.
Earth Lab Graduate Students
Our dedicated graduate students work hard to contribute to Earth Lab's body of research while getting their degrees. This month, we'd like to recognize their work.
Biodiversity monitoring is essential for conservation. However, wildlife surveys are resource-intensive and riddled with observation error. Scientists use statistical methods with these limited datasets to make target inferences, like occupancy models to estimate population dynamics. However, most occupancy models do not account for taxonomic uncertainty, which leads to biased estimates of ecologically meaningful measurements. We addressed this knowledge gap by building a joint occupancy-classification model that quantifies the probability that an organism was correctly identified in a multispecies survey dataset. We illustrate our model using the NEON carabid dataset at Niwot Ridge, CO as a case study. This is the first modeling framework to find that occupancy rates can inform species classification. The manuscript, in review at Methods in Ecology & Evolution, is available on bioRxiv.
How are forests recovering after wildfire? We are using images from drones, airplanes, and satellites to study post-fire forest composition (which species are present) and carbon storage changes over time. We recently published a method to link field-based tree measurements with airborne remote sensing images to classify tree species using freely available National Ecological Observatory Network data. We also participated in a data science competition (https://idtrees.org/) to develop plant identification techniques that transfer across sites. We are building upon these methods to map carbon storage in forests as they recover after wildfires, starting with drone flights this summer.
Related publication: Remote Sensing Special Issue, She Maps
Here at Earth Lab, I am involved in a few projects. For one project, we developed an algorithm that gets gridded fire data from the internet which then creates fire perimeters. Right now it works for the United States, and we are trying to make it work for the entire Earth. Another project I'm working on is using the FIRED data to understand whether fires are burning faster, and what this means for the future. We are also using FIRED with other satellite data to better understand how warming climate alters the way fires behave at night.
Related Publication: Remote Sensing Special Issue, Earth Observations for Ecosystem Resilience